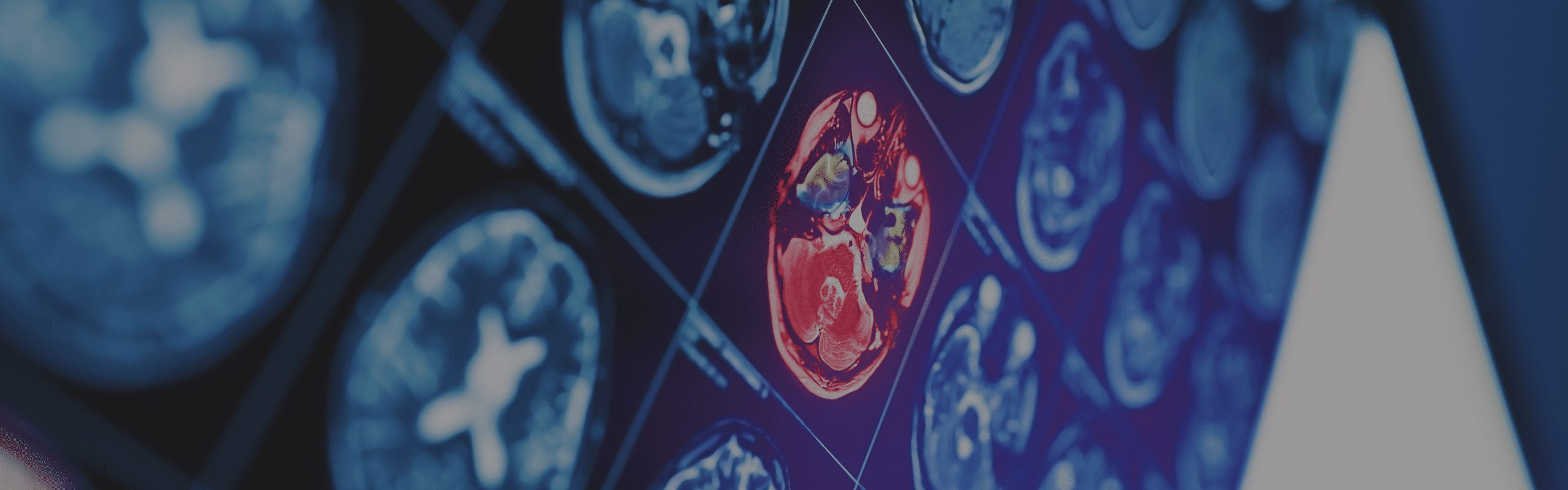
Workstations for AI and High Performance Computing
Process large amounts of data quickly and efficiently with our powerful systems. Handle large language models, complex simulations, and data analysis with ease.
At Puget Systems, our goal is to make purchasing and owning computers a pleasure, not a hindrance to your work. We know that scientific computing requires high-performing workstations that can handle the unique demands of computationally intensive algorithms. Thankfully we have an expert in this field on staff: Dr. Don Kinghorn. He spends a lot of time testing the capabilities of new computer hardware, recently focusing on large language models, as well as writing informational posts in his HPC Blog and assisting our consulting team with customer questions. He has helped tailor our science workstation configurations for various aspects of research, data processing, and machine learning using high-performance processors, multiple GPUs, or a mix of both.
Our Customers Include
View more of our customers here.
High-Performance Workstations for Cutting-Edge Research and Computational Tasks
At Puget Systems, our powerful workstation PCs for scientific computing are crafted through a combination of our on-staff experts, their benchmark testing, customer feedback, and the knowledge our consulting team has accumulated over the years.
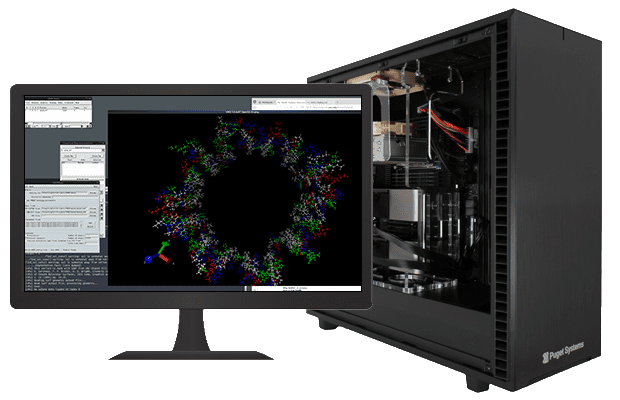
Select Your Workflow
Choose your workflow below. If you have questions about what type of computer hardware your specific research needs, our expert consultants are available to provide individualized guidance or a quote for a custom scientific computing workstation.
Or Shop by Hardware
Talk to an Expert
Are you a scientist, researcher, or engineer looking for a high-performance PC to handle your computational needs? We can help! Here at Puget Systems, we specialize in building custom workstations tailored specifically to meet the unique demands of scientific computing. We understand that every client has different requirements, so we have highly knowledgeable, non-commissioned consultants available to discuss your specific situation. They can work with you every step of the way to configure a computer that meets your individual needs, whether you require a computer for machine learning, inference, data analysis, simulations, or other tasks. There is no cost or obligation, and we promise a no-pressure experience. So, if you’re looking for a scientific workstation that can handle your computational needs, start a conversation with us today using whichever of these methods works best for you.
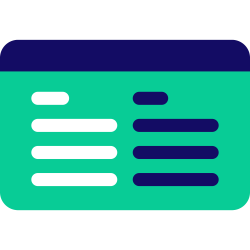
Request a Consultation by Email
We know you are busy, so you may find that the most convenient option to get started with a consultation is via email. Just click the button below and fill in your name, address, and some information about what you are looking for and we’ll get back to you within one business day.
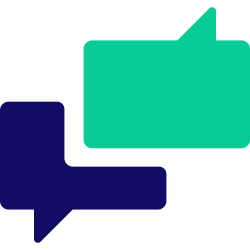
Schedule a Phone Consultation
If you would prefer to talk directly with a consultant, rather than go back and forth via email, we have a scheduling system where you can select the day and time that would be best for us to give you a call. Click the button below to book your time.
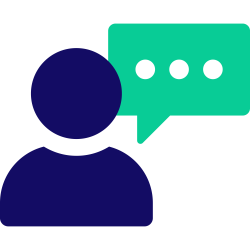
Give Us a Call
If you’d rather not wait, you can reach out to us via phone during our business hours.
Monday – Friday | 7am – 5pm (Pacific)
425-458-0273 OR 1-888-PUGETPC (784-3872)