Table of Contents
Introduction (and a bit of history!)
In this post I've done more testing with Ryzen 3900X looking at the effect of BLAS libraries on a simple but computationally demanding problem with Python numpy. The results may surprise you! I start with a little bit of history of Intel vs AMD performance to give you what may be a new perspective on the issue.
More reality check with the AMD Zen2 Ryzen 3900X! There are two necessary and equally important ingredients needed to do anything useful with a computer, the hardware and the software.
The hardware and software together with helpful tools like special performance/feature libraries, and community support when made available for developers and the users, in an organized, cohesive, professional and easily usable manner make up what is referred to as an "Ecosystem".
The best example (ever!), of a well done hardware/software ecosystem is what NVIDIA has done with GPU accelerated computing.
On the CPU side of computing, Intel has a very good, and lately, rapidly expanding, ecosystem for the x86 CPU architecture. Intel learned some lessons from the glory days of the proprietary UNIX hardware vendors. The UNIX hardware vendors all had their own proprietary compilers and numerical libraries i.e. BLAS libraries. When the UNIX hardware/software goliath, DEC (Digital Equipment Corporation), shutdown some of their talented compiler developers went to Intel (Important VMS OS devs went to Microsoft and created WindowsNT). Intel developed a "best in class" compiler suite and the highly optimized compute library collection MKL (Math Kernel Library).
What about AMD? AMD is the only other company to have license to the x86 architecture. Indeed AMD is responsible for many advancements to the arch, like 64-bit x86_64. … which was originally known as AMD64! (amd64 is still used as the name extension for 64-bit x86 Linux binary packages). So, do the Intel compilers and MKL work with AMD CPU's? Yes, kind of…
When Intel first released their (fantastic) compilers and MKL it was soon discovered that they worked for AMD processors but didn't give very good performance (even though the core architecture was the same). Someone got the idea to spoof the processor ID (hard to do) on an AMD Opteron so that it responded as "Genuine Intel" sure enough the performance went way up! When program calls to MKL start up the first thing that happens is a check for "Genuine Intel" and the compute-core-features available. Then the calls execute a "code-path" with the best optimizations for the core features. But, if the ID is AMD then the code-path chosen is an old SSE optimization path, i.e. no modern performance optimizations. Intel has every right to do that! It IS their stuff, and there IS some incompatibility at the highest (or lowest) levels of optimization for the hardware. And, MKL is insanely well optimized for Intel CPU's … as it should be!
Long story, short, there was a lawsuit and a settlement. Nothing much changed except that Intel has to include an "Optimization notice" on their publications.
The bottom line is that, Intel is a massive company compared to AMD and their resources for developing an "ecosystem" are much greater. AMD is doing good work on their ecosystem. There is an optimizing compiler project, AAOC and AMD's BLIS (BLAS) performance library.
To get the best out of AMD hardware you often have to do a little extra work. It's good to be aware of that! The new AMD hardware is looking really good and they have gotten a very important contract for what will be the US's first ExaScale Supercomputer. That DOE contract will provide a large amount of funding and highly skilled developers to expand and optimize the "ecosystem" for AMD. It will definitely be getting better …soon!
Test systems: AMD Ryzen 3900X and Intel Xeon 2175W
AMD Hardware
- AMD Ryzen 3900X 12-core AVX2
- Motherboard Gigabyte X570 AORUS ULTRA
- Memory 4x DDR4-3200 16GB (64GB total)
- 2TB Intel 660p NVMe M.2
- NVIDIA 2080Ti GPU
Intel Hardware
- Intel Xeon-W 2175 14-core AVX512
- ASUS C422 Pro SE (My personal workstation )
- 128GB DDR4 2400 MHz Reg ECC memory
- Samsung 960 EVO 1TB NVMe M.2
- NVIDIA Titan V GPU
Software
- Ubuntu 18.04
- Anaconda Python build Anaconda3-2019.07-Linux-x86_64
- numpy 1.16.4 (default env)
- mkl 2019.4 (default env)
- libopenblas 0.3.6 (in my "openblas-np env)
I will describe how to create an env with numpy linked to OpenBLAS in the section after the results.
Notes:
- OpenBLAS is an excellent open source BLAS library based on the, highly regarded, work originally done by Kazushige Goto.
- OpenBLAS does not currently have optimizations for AVX512 (It does include AVX2 optimizations)
Now onto some simple testing that will illustrate the consequences of the history discussed in the introduction.
Ryzen 3900X and Xeon 2175W performance using MKL and OpenBLAS for a Python numpy “norm of matrix product” calculation
numpy is the most commonly used numerical computing package in Python. The calculation presented in this testing is very simple but computationally intensive. It will take advantage of the BLAS library that gives numpy it's great performance. In this case we will use Anaconda Python with "envs" setup for numpy linked with Intel MKL (the default) and with OpenBLAS (described in the next section).
Those are pretty dramatic differences! The standout features are,
- MKL provides tremendous performance optimization on Intel CPU's The test job is definitely benefiting from AVX512 optimizations which are not available in this OpenBLAS version.
- OpenBLAS levels the performance difference considerably by providing good optimization up to the level of AVX2. (keep in mind that the 2175W is 14-core vs 12-cores on the Ryzen 3900X)
- The low optimization code-path used for AMD CPU's by MKL is devastating to performance.
This test clearly shows the effect of hardware specific code optimization. It is also pretty synthetic! In the real world programs are more complicated and are usually not anywhere near fully optimized especially in regards to vectorization that takes advantage of AVX. There are also common numerical libraries that are not so heavily targeted to specific architectures. For example, the popular, and very good, C++ boost library suite.
Note: I also tried to setup a numpy linked with AMD BLIS lib but it did not work correctly (very poor performance). I did not troubleshoot the issues.
Creating an “env” with conda that includes OpenBLAS for numpy
What I did to get numpy with different BLAS lib links in Anaconda python was simple.
Create and activate an env for the OpenBLAS linked numpy,
conda create --name openblas-np
conda activate openblas-np
Then install numpy specifying the BLAS library,
conda install numpy jupyter ipykernel blas=*=openblas
Then I created a kernel for Jupyter notebook and started a notebook using that kernel,
python -m ipykernel install --user --name openblas-np
Following is the Jupyter notebook input cells for the test,
import numpy as np
import time
n = 20000
A = np.random.randn(n,n).astype('float64')
B = np.random.randn(n,n).astype('float64')
start_time = time.time()
nrm = np.linalg.norm(A@B)
print(" took {} seconds ".format(time.time() - start_time))
print(" norm = ",nrm)
Here's a command and the output that shows that the numpy configuration is indeed using OpenBLAS,
np.__config__.show()
blas_mkl_info:
NOT AVAILABLE
blis_info:
NOT AVAILABLE
openblas_info:
libraries = ['openblas', 'openblas']
library_dirs = ['/home/kinghorn/anaconda3/envs/openblas-np/lib']
language = c
define_macros = [('HAVE_CBLAS', None)]
blas_opt_info:
libraries = ['openblas', 'openblas']
library_dirs = ['/home/kinghorn/anaconda3/envs/openblas-np/lib']
language = c
define_macros = [('HAVE_CBLAS', None)]
lapack_mkl_info:
NOT AVAILABLE
openblas_lapack_info:
libraries = ['openblas', 'openblas']
library_dirs = ['/home/kinghorn/anaconda3/envs/openblas-np/lib']
language = c
define_macros = [('HAVE_CBLAS', None)]
lapack_opt_info:
libraries = ['openblas', 'openblas']
library_dirs = ['/home/kinghorn/anaconda3/envs/openblas-np/lib']
language = c
define_macros = [('HAVE_CBLAS', None)]
Conclusions
When I started this testing I was expecting to see the results like that I found, however, I was still surprised when I saw them! I hope this post has given you a new perspective on the AMD vs Intel thing. I have great respect for both of these companies and I know we are going to see good things from both of them going forward.
The AMD Ryzen Zen2 processors are impressive and seem to be an excellent value. We are working diligently on validating full platforms. We are not rushing this processes. There are still rough edges on the total system package that we want to get right. I am looking forward to getting my hands on the next Threadripper and hopefully will get a chance to fire up an Epyc Rome system too.
Happy computing! –dbk @dbkinghorn
Puget Systems offers a range of powerful and reliable systems that are tailor-made for your unique workflow.
Related Content
Why Choose Puget Systems?
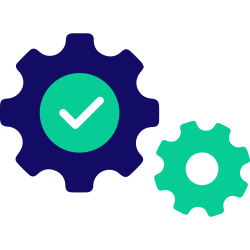
Built Specifically for You
Rather than getting a generic workstation, our systems are designed around your unique workflow and are optimized for the work you do every day.
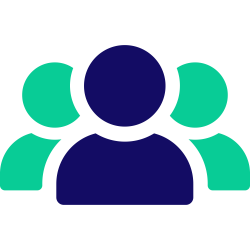
We’re Here, Give Us a Call!
We make sure our representatives are as accessible as possible, by phone and email. At Puget Systems, you can actually talk to a real person!
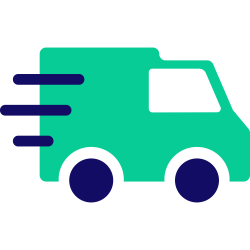
Fast Build Times
By keeping inventory of our most popular parts, and maintaining a short supply line to parts we need, we are able to offer an industry-leading ship time.
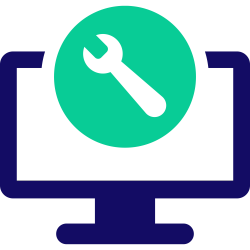
Lifetime Labor & Tech Support
Even when your parts warranty expires, we continue to answer your questions and even fix your computer with no labor costs.
Click here for even more reasons!