Table of Contents
Introduction
This is a short post showing a performance comparison with the RTX2070 Super and several GPU configurations from recent testing. The comparison is with TensorFlow running a ResNet-50 and Big-LSTM benchmark.
I was at Puget Systems Labs when our inventory manager came in with an RTX2070 Super and said "this thing has an NVLINK connector, you might be interested in that" … Nice! I was indeed interested. I immediately thought, two of these could be a great relatively inexpensive setup for ML/AI work. That thought was echoed back to me from someone commenting on one of my blog posts who was thinking the same thing. I replied, I was eager to try it. I finally got some time in with two of these nice GPU's and an NVLINK bridge.
Here's the testbed,
System Configuration
Hardware:
- Intel Core i9 9960X (16-core)
- Gigabyte X299 Designare EX
- 8x DDR4-2666 16GB (128GB total)
- Intel 2TB 660p NVMe M.2
- 2 x NVIDIA RTX 2070 Super + NVLINK Bridge
Software:
- Ubuntu 18.04
- NVIDIA display driver 430.40 (from Graphics-Drivers ppa)
- Docker 19.03.0-ce
- NVIDIA-Container-Toolkit 1.0
- NVIDIA NGC container registry
- Container image: nvcr.io/nvidia/tensorflow:19.02-py3 for "Big LSTM" and "CNN"
NOTE: I have updated my Docker-ce setup to the latest version and am now using the native "nvidia-container-runtime". I will be writing a post with detailed instructions for this new configuration soon. My older posts have used NVIDIA-Docker2 which is now deprecated.
I used the NGC TensorFlow container tagged 19.02-py3 for consistency with other results in the charts below.
Results
The older results in these charts are from the more extensive testing in the post TensorFlow Performance with 1-4 GPUs — RTX Titan, 2080Ti, 2080, 2070, GTX 1660Ti, 1070, 1080Ti, and Titan V. Please see that link for detailed information about the job run command-line arguments etc..
[ResNet-50 fp32] TensorFlow, Training performance (Images/second) comparison using 2 NVIDIA RTX 2070-Super GPU’s
These results show the RTX2070-Super performing as well as the 2080's. The multi-GPU methodology is using "Horovod" i.e. MPI for data-parallel scaling so there is little effect from using the NVLINK bridge. An interesting thing to note is that for this job the performance with 2 x 2070-Super's is slightly better than a single RTX Titan.
[ResNet-50 fp16 Tensor-cores] TensorFlow, Training performance (Images/second) comparison using 2 NVIDIA RTX 2070-Super GPU’s
The 2070-Super did very well at fp16.
TensorFlow LSTM: Big-LSTM 1 Billion Word Dataset
With this recurrent neural network there is more GPU-GPU communication so NVLINK has more impact. The 2 x 2070-Super + NVLINK configuration did a little better than a single RTX Titan.
Conclusions
It looks like the NVIDIA RTX2070 Super is indeed a great GPU! At $500 it seems like a bargain and is worth considering for a ML/AI setup. The performance was near that of the RTX 2080 and in a dual configuration it performed as well as the RTX Titan, at least for the limited testing in this post.
The RTX2070 Super tested were the NVIDIA editions and had the side fans which I really don't care for, (they blow hot air into the case instead of out the back and are easily obstructed). I expect that there will be other vendor releases of these cards that use the blower style fans which is a much better cooling solution for GPU's that will be under heavy load.
My favorite workstation GPU for ML/AI work right now is the RTX2080Ti. The 12GB of memory on the 2080Ti is a big plus over the 8GB on the RTX2070 Super. If you have input data with a large number of features you may hit the dreaded OOM (Out of Memory) with an 8GB GPU. However, having 2 of the 2070-Super's for less cost than a single 2080Ti is very tempting and definitely worth considering if the 8GB on the 2070 Super will suffice for your work!
Happy computing! –dbk @dbkinghorn
Appendix: Peer to Peer Bandwidth and Latency results for 2 RTX 2070 Super GPU’s
The RTX 2070Super has a single sub-link (like the RTX 2080). The RTX 2080Ti and RTX Titan (as well as the RTX Quadro's) have dual sub-links. This means that the 2070-Super has 1/2 the NVLINK bandwidth as the 2080Ti and RTX Titan.
kinghorn@utest:~/projects/samples-10.0/bin/x86_64/linux/release$ ./p2pBandwidthLatencyTest
[P2P (Peer-to-Peer) GPU Bandwidth Latency Test]
Device: 0, Graphics Device, pciBusID: a1, pciDeviceID: 0, pciDomainID:0
Device: 1, Graphics Device, pciBusID: c1, pciDeviceID: 0, pciDomainID:0
Device=0 CAN Access Peer Device=1
Device=1 CAN Access Peer Device=0
***NOTE: In case a device doesn't have P2P access to other one, it falls back to normal memcopy procedure.
So you can see lesser Bandwidth (GB/s) and unstable Latency (us) in those cases.
P2P Connectivity Matrix
DD 0 1
0 1 1
1 1 1
Unidirectional P2P=Disabled Bandwidth Matrix (GB/s)
DD 0 1
0 386.90 5.82
1 5.83 387.81
Unidirectional P2P=Enabled Bandwidth (P2P Writes) Matrix (GB/s)
DD 0 1
0 387.93 24.23
1 24.25 371.77
Bidirectional P2P=Disabled Bandwidth Matrix (GB/s)
DD 0 1
0 388.80 11.63
1 11.69 387.52
Bidirectional P2P=Enabled Bandwidth Matrix (GB/s)
DD 0 1
0 388.58 48.38
1 48.38 377.05
P2P=Disabled Latency Matrix (us)
GPU 0 1
0 1.49 11.40
1 11.27 1.56
CPU 0 1
0 2.96 7.67
1 7.17 2.87
P2P=Enabled Latency (P2P Writes) Matrix (us)
GPU 0 1
0 1.49 0.89
1 0.90 1.56
CPU 0 1
0 2.95 2.00
1 2.05 2.88
Puget Systems offers a range of powerful and reliable systems that are tailor-made for your unique workflow.
Related Content
Why Choose Puget Systems?
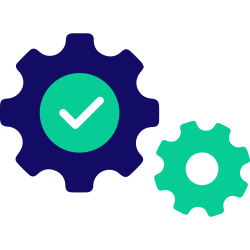
Built Specifically for You
Rather than getting a generic workstation, our systems are designed around your unique workflow and are optimized for the work you do every day.
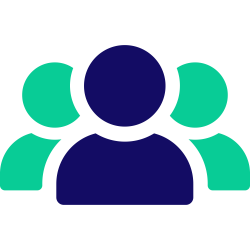
We’re Here, Give Us a Call!
We make sure our representatives are as accessible as possible, by phone and email. At Puget Systems, you can actually talk to a real person!
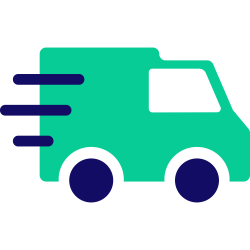
Fast Build Times
By keeping inventory of our most popular parts, and maintaining a short supply line to parts we need, we are able to offer an industry-leading ship time.
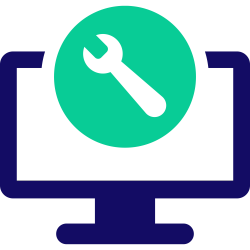
Lifetime Labor & Tech Support
Even when your parts warranty expires, we continue to answer your questions and even fix your computer with no labor costs.
Click here for even more reasons!